F# Math (IV.) - Writing generic numeric code
Generic numeric code is some calculation that can be used for working with multiple different numeric
types including types such as int
, decimal
and float
or even our own
numeric types (such as the type for clock arithmetic
from the previous article of the series). Generic numeric code differs from ordinary generic F# code such
as the 'a list
type or List.map
function, because numeric code uses numeric operators
such as +
or >=
that are defined differently for each numeric type.
When writing simple generic code that has some type parameter 'T
, we don’t know anything about
the type parameter and there is no way to restrict it to a numeric type that provides all the operators that
we may need to use in our code. This is a limitation of the .NET runtime and F# provides two ways for
overcoming it.
- Static member constraints can be used to write generic code where the actual
numeric operations are resolved at compile-time (and a generic function is specialized for all required
numeric types). This approach makes resulting code very efficient and is generally very easy to use
when writing a function such as
List.sum
. - Global numeric associations (available in F# PowerPack) give us a way to obtain
an interface implementing required numeric operations dynamically at runtime. This approach has some
runtime overhead, but can be used for complex numeric types (such as
Matrix<'T>
). - Combination of both techniques can be used to implement complex numeric type that is generic over the contained numeric values and has only a minimal runtime overhead.
Static member constraints are a unique feature of F# that is not available in other .NET languages, so if you're interested in writing numeric code for .NET, this may be a good reason for choosing F#. In C# or Visual Basic, you would be limited to the second option (which can be implemented in C#). In dynamic languages (like IronPython), everything is dynamic, so numeric computations can work with any numeric type, but will be significantly less efficient. In the rest of the article, we look at the three options summarized above.
This article is a part of a series that covers some F# and F# PowerPack features for numerical computing. Other articles in this series discuss matrices, defining custom numeric types and writing generic code. For links to other parts, see F# Math - Overview of F# PowerPack.
Published: Sunday, 27 November 2011, 5:19 PM
Tags:
c#, functional, f#, math and numerics
Read the complete article
F# Math (III.) - Defining custom numeric types
In this article, we define an F# numeric type for calculating in the modular arithmetic (also called clock arithmetic) [1]. Modular arithmetic is used for calculations where we want to keep a value within a specified range by counting in cycles. For example, a maximal value on clock is 12 hours. When we add 11 hours and 3 hours, the value overflows and the result is 2 hours. Aside from clocks, this numeric system is also essential in cryptography or, for example, in music.
This tutorial shows several techniques that are essential when defining any new numeric type in F#. Most importantly, you’ll learn how to:
- Define a numeric type with overloaded operators
- Define a numeric literal for constructing numbers of our new type
- Enable calculating with our type in F# lists and matrices
- Hide implementation details of a numeric type
We define type IntegerZ5
that implements modular arithmetic with modulus 5, meaning that valid values are in the range
from 0 to 4 and we equip the type with operations such as addition and multiplication. When an operation produces a value that would
be outside of the range, we adjust it by adding or subtracting the modulus (in our case 5). Here are some examples of calculations
that we’ll be able to write:
2 + 1 = 3 (mod 5) 4 * 2 = 3 (mod 5) List.sum [ 0; 1; 2; 3 ] = 1 (mod 5)
In the first case, we can perform the operation without any adjustments. In the second case, we multiply 4 by 2 and get 8 as the
result, which is out of the required range. To correct it, we calculate the remainder after a division by 5 (written as 8 % 5
in F#),
which gives us 3. Finally, the last example shows that we’d also like to be able to use our type with lists. If we add values 0, 1, 2
and 3, we get 6 which is adjusted to 1.
This article is a part of a series that covers some F# and F# PowerPack features for numerical computing. Other articles in this series discuss matrices, defining custom numeric types and writing generic code. For links to other parts, see F# Math - Overview of F# PowerPack.
Published: Thursday, 24 November 2011, 7:21 PM
Tags:
functional, f#, math and numerics
Read the complete article
F# Math (II.) - Using matrices for graph algorithms
In the previous article of this
series, we looked at complex
and BigRational
, which are two
numeric types that are available in F# PowerPack. Aside from these two, the PowerPack
library also contains a type matrix
representing a two-dimensional matrix
of floating-point values.
In this article, you'll learn how to work with matrices in F#, using some of the functions provided by F# PowerPack. I'll demonstrate the library using an example that represents graphs using a, so called, adjacency matrix. If you're not familiar with this concept, you don't need to worry. It is quite simple and it will be clear once we look at an example. The matrix represents which vertices of a graph are connected with other vertices by an edge. Many of the standard operations on matrices are useful when working with adjacency matrix, so this tutorial will cover the following:
- Creating matrices from lists and using functions from the
Matrix
module - Using slices to read or modify a part of matrix
- Performing standard operations with matrices such as transposition and matrix multiplication
- Using higher order functions for working with matrices
This article is a part of a series that covers some F# and F# PowerPack features for numerical computing. Other articles in this series discuss matrices, defining custom numeric types and writing generic code. For links to other parts, see F# Math - Overview of F# PowerPack.
Published: Wednesday, 9 November 2011, 1:46 AM
Tags:
functional, f#, math and numerics
Read the complete article
F# Math (I.) - Numeric types in PowerPack
In this article, we'll briefly look at two numeric types that are available in F# PowerPack.
The type complex
represents complex numbers consisting of real and imaginary parts.
Both parts are stored as a floating point numbers. The type BigRational
represents
rational numbers consisting of numerator and denominator of arbitrary sizes. Integers of arbitrary
size are represented using BigInteger
type that is available in .NET 4.0
(in the System.Numerics.dll
assembly). On .NET 2.0, the BigInteger
type is also a part of F# PowerPack.
This article is a part of a series that covers some F# and F# PowerPack features for numerical computing. Other articles in this series discuss matrices, defining custom numeric types and writing generic code. For links to other parts, see F# Math - Overview of F# PowerPack.
Published: Wednesday, 2 November 2011, 2:34 AM
Tags:
c#, functional, f#, math and numerics
Read the complete article
F# Math - Numerical computing and F# PowerPack
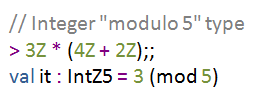
This article is the first article of a series where I'll explain some of the F# features that are useful for numeric computing as well as some functionality from the F# PowerPack library. Most of the content was originally written for the Numerical Computing in F# chapter on MSDN (that I announced earlier), but then we decided to focus on using F# with third party libraries that provide more efficient implementation and richer set of standard numeric functionality that's needed when implementing machine learning and probabilistic algorithms or performing statistical analysis. If you're interested in these topics, then the last section (below) gives links to the important MSDN articles.
However, F# PowerPack still contains some useful functionality. It includes two additional numeric types and an implementation of matrix that integrates nicely with F#. The series also demonstrates how to use features of the F# language (and core libraries) to write numeric code elegantly. In particular, we'll use the following aspects:
- Overloaded operators. Any type can provide overloaded version of standard numeric operators such as +, -, * and / as well as other non-standard operators (such as .*). As a result, libraries can implement their own numeric types which are indistinguishable from built-in types such as int.
- Numeric literals. F# math libraries can enable using new numeric literals in the code. For example, you can
write a
BigRational
value representing one third as1N/3N
. TheN
suffix used in the notation is not hardcoded in the F# language and we'll see how to define similar numeric type. - Static constraints. F# supports static member constraints, which can be used for writing functions that
work with any numeric type. For example, the
List.sum
function uses this feature. It can sum elements of any list containing numbers.
These are just a few of the F# language features that are useful when writing numeric code, but there are many others. The usual F# development style using interactive tools, type safety that prevents common errors, units of measure as well the expressivity of F# make it a great tool for writing numeric code. For more information, take a look at the MSDN overview article Writing Succinct and Correct Numerical Computations with F#.
Published: Wednesday, 2 November 2011, 2:30 AM
Tags:
functional, f#, writing, math and numerics
Read the complete article
Accelerator and F# (IV.): Composing computations with quotations
In this article series, we're talking about the Accelerator project and I'm
presenting an F# library that I implemented, which allows you to use Accelerator [references]
in a more sophisticated way. We've seen two examples of using Accelerator directly
(see also introduction and Game of Life).
In the previous article
I introduced my F# library for working with Accelerator. We've seen F# functions from the
DataParallel
module, we implemented an algorithm that rotates an image
using these functions and finally, we've seen that we can take this ordinary F# code
and run it using Accelerator. This is all possible thanks to F# quotations, which
we can use to get an AST (a source code) of an F# function we wrote (if the function
is marked in some special way).
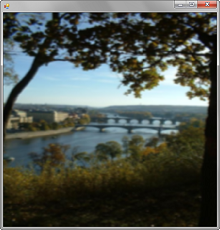
In this part of the series, we're going to look at working with quotations explicitly. We'll use meta-programming techniques to work with Accelerator. Meta-programming means writing programs that manipulate with other programs or pieces of code. This is exactly what we're going to do in this article. We'll write an F# function (running on CPU) that builds a program, which we'll then run using Accelerator.
This is quite interesting approach, which isn't possible when we call Accelerator methods as standard F# functions or .NET methods. The benefit is that we'll clearly see which parts of program run on CPU and what parts execute on GPU or using X64 multi-core target. We could also perform more complicated optimizations with the code (because this wouldn't affect the readability). Just for your reference, here is the list of articles in this series in case you missed some of them:
- Accelerator and F# (I.): Introduction and calculating PI
- Accelerator and F# (II.): The Game of Life on GPU
- Accelerator and F# (III.): Data-parallel programs using F# quotations
- Accelerator and F# (IV.): Composing computations with quotations
However, enough with theory and let's take a look at some code samples! This time, we'll implement blurring of an image (also called convolution). Another example how to write this in F# using Accelerator is Satnam Singh's blog post [4]. Our example will be different, because we'll write the code as standard F# program and then have it translated to Accelerator automatically using quotations. We'll also talk about the architecture of the library that we're using and look at some performance results.
Published: Tuesday, 12 January 2010, 3:20 AM
Tags:
functional, academic, meta-programming, accelerator, f#, math and numerics
Read the complete article
Accelerator and F# (III.): Data-parallel programs using F# quotations
If you've been following this article series, you already know that Accelerator is a MSR library [1, 2] that allows you to run code in parallel on either multi-core CPU or using shaders on GPU (see introduction). We also discussed a direct way to use Accelerator from F# (by calling Accelerator methods directly) and implemented Conway's Game of Life. In this article, we'll look at more sophisticated way of using Accelerator from F#. We'll introduce F# quotations and look at translating 'normal' F# code to use Accelerator.
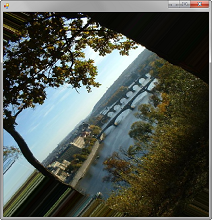
In general, F# quotations allow us to treat F# code as data structure and manipulate with it. This is very similar to C# expression trees, but the F# implementation is more powerful. We can also mark a standard method or a function with a special attribute that tells the compiler to store quotation of the body. Then we can access the quotation and traverse it or modify it. In this article we'll use a function that takes an F# quotation (containing a limited set of functions) and executes it using MSR Accelerator. Implementing this functionality is a bit complicated, so we won't discuss the implementation now. We'll leave this for some future article of this series. In future, we'll also look at other interesting possibilities that we have when writing code using quotations. Here is a list of articles in this series and of the articles that I'm planning to add:
- Accelerator and F# (I.): Introduction and calculating PI
- Accelerator and F# (II.): The Game of Life on GPU
- Accelerator and F# (III.): Data-parallel programs using F# quotations
- Accelerator and F# (IV.): Composing computations with quotations
Published: Monday, 4 January 2010, 12:50 PM
Tags:
academic, functional, meta-programming, accelerator, f#, math and numerics
Read the complete article
Accelerator and F# (II.): The Game of Life on GPU
In the previous article, I introduced the Microsoft Research Accelerator library. It allows us to write computations with arrays in C# and execute them in parallel on multi-core CPU or more interestingly, using GPU shaders. In the previous artcile, we've seen how Accelerator works and how it can be accessed from F#. In this article, we'll look at one more interesting F# demo - we'll implement the famous Conway's Game of Life [1] using Accelerator. We'll use a v2 version of Accelerator which has been announced just recently and is available from Microsoft Connect [2].
This article is the second one from a series about using Accelerator from F#. Today, we'll use Accelerator types directly from F# - this is the simplest possible approach and is very similar to the way you'd work with Accelerator in C#. However, we can use some nice F# features such as custom operators to make the code more readable. In the next article, we'll discuss a different approach - we'll look how to execute more "standard" F# code (that doesn't reference Accelerator explicitly) with Accelerator using F# quotations. The list of articles may change, but here is a list of articles that I'm currently planning to write:
- Accelerator and F# (I.): Introduction and calculating PI
- Accelerator and F# (II.): The Game of Life on GPU
- Accelerator and F# (III.): Data-parallel programs using F# quotations
- Accelerator and F# (IV.): Composing computations with quotations
Published: Monday, 28 December 2009, 9:16 PM
Tags:
academic, functional, meta-programming, accelerator, f#, math and numerics
Read the complete article
Accelerator and F# (I.): Introduction and calculating PI
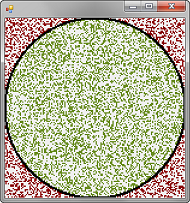
I already wrote about two projects that I worked on during an internship at MSR back in 2007 (ASP.NET support in F# and F# WebTools). Even though this was more than 2 years ago (and I did one more internship at MSR in the meantime), I still have one more project that I never published on the web. The folks from the F# team reminded me of this project recently, so I thought I could finally publish it. The project used Microsoft Research Accelerator [1, 2], which is a C# library for developing array-based computations and executing them on a GPU. More recently, the Accelerator team at MSR published Accelerator v2 [3], which was a good motivation to update my original project...
In this article, we'll look at the simplest way of using Accelerator from F#. Accelerator provides a managed interface that can be naturally used from both C# and F#. We can use a mix of method calls and overloaded operators to describe a computation. In F#, we'll also define our additional custom operators to make the code a bit nicer. After we introduce Accelerator using a simple C# demo, we'll look how to calculate an approximate value of the PI number using a Monte-Carlo method.
This article is the first one from a series about using Accelerator from F#. The list of articles may change, but here is a list of articles that I'm currently planning to write:
- Accelerator and F# (I.): Introduction and calculating PI
- Accelerator and F# (II.): The Game of Life on GPU
- Accelerator and F# (III.): Data-parallel programs using F# quotations
- Accelerator and F# (IV.): Composing computations with quotations
Published: Monday, 21 December 2009, 3:21 AM
Tags:
functional, academic, meta-programming, accelerator, f#, math and numerics
Read the complete article